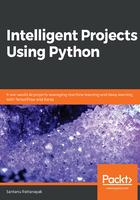
Q-learning
We will now look at a popular reinforcement learning algorithm, called Q-learning. Q-learning is used to determine an optimal action selection policy for a given finite Markov decision process. A Markov decision process is defined by a state space, S; an action space, A; an immediate rewards set, R; a probability of the next state, S(t+1), given the current state, S(t); a current action, a(t); P(S(t+1)/S(t);r(t)); and a discount factor, . The following diagram illustrates a Markov decision process, where the next state is dependent on the current state and any actions taken in the current state:

Let's suppose that we have a sequence of states, actions, and corresponding rewards, as follows:

If we consider the long term reward, Rt, at step t, it is equal to the sum of the immediate rewards at each step, from t until the end, as follows:

Now, a Markov decision process is a random process, and it is not possible to get the same next step, S(t+1), based on S(t) and a(t) every time; so, we apply a discount factor, , to future rewards. This means that, the long-term reward can be better represented as follows:

Since at the time step, t, the immediate reward is already realized, to maximize the long-term reward, we need to maximize the long-term reward at the time step t+1 (that is, Rt+1), by choosing an optimal action. The maximum long-term reward expected at a state S(t) by taking an action a(t) is represented by the following Q-function:

At each state, s ∈ S, the agent in Q-learning tries to take an action, , that maximizes its long-term reward. The Q-learning algorithm is an iterative process, the update rule of which is as follows:

As you can see, the algorithm is inspired by the notion of a long-term reward, as expressed in (1).
The overall cumulative reward, Q(s(t), a(t)), of taking action a(t) in state s(t) is dependent on the immediate reward, r(t), and the maximum long-term reward that we can hope for at the new step, s(t+1). In a Markov decision process, the new state s(t+1) is stochastically dependent on the current state, s(t), and the action taken a(t) through a probability density/mass function of the form P(S(t+1)/S(t);r(t)).
The algorithm keeps on updating the expected long-term cumulative reward by taking a weighted average of the old expectation and the new long-term reward, based on the value of .
Once we have built the Q(s,a) function through the iterative algorithm, while playing the game based on a given state s we can take the best action, , as the policy that maximizes the Q-function:
