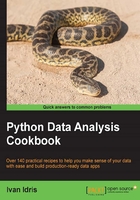
Introduction
Reproducible data analysis is a cornerstone of good science. In today's rapidly evolving world of science and technology, reproducibility is a hot topic. Reproducibility is about lowering barriers for other people. It may seem strange or unnecessary, but reproducible analysis is essential to get your work acknowledged by others. If a lot of people confirm your results, it will have a positive effect on your career. However, reproducible analysis is hard. It has important economic consequences, as you can read in Freedman LP, Cockburn IM, Simcoe TS (2015) The Economics of Reproducibility in Preclinical Research. PLoS Biol 13(6): e1002165. doi:10.1371/journal.pbio.1002165.
So reproducibility is important for society and for you, but how does it apply to Python users? Well, we want to lower barriers for others by:
- Giving information about the software and hardware we used, including versions.
- Sharing virtual environments.
- Logging program behavior.
- Unit testing the code. This also serves as documentation of sorts.
- Sharing configuration files.
- Seeding random generators and making sure program behavior is as deterministic as possible.
- Standardizing reporting, data access, and code style.
I created the dautil
package for this book, which you can install with pip or from the source archive provided in this book's code bundle. If you are in a hurry, run $ python install_ch1.py
to install most of the software for this chapter, including dautil
. I created a test Docker image, which you can use if you don't want to install anything except Docker (see the recipe, Sandboxing Python applications with Docker images).